The meaning of a "negative" result in food microbiological testing varies depending on the sampling plan used. This article aims to explain the sampling plans of the International Commission on Microbiological Specifications for Foods (ICMSF) in an accessible manner. These sampling plans are adopted in the EU's food safety and process hygiene standards. Understanding the rationale behind these sampling plans is more important than memorising the plans themselves. This article focuses on explaining the "why" for beginners.
The Meaning of "Negative" in Food Microbiological Testing
When a negative result for Salmonella is reported for a batch of food, it’s important to consider what "negative" actually means.
- Was only one sample tested and found negative?
- Were five samples tested and all negative?
- Were 30 samples tested and all negative?
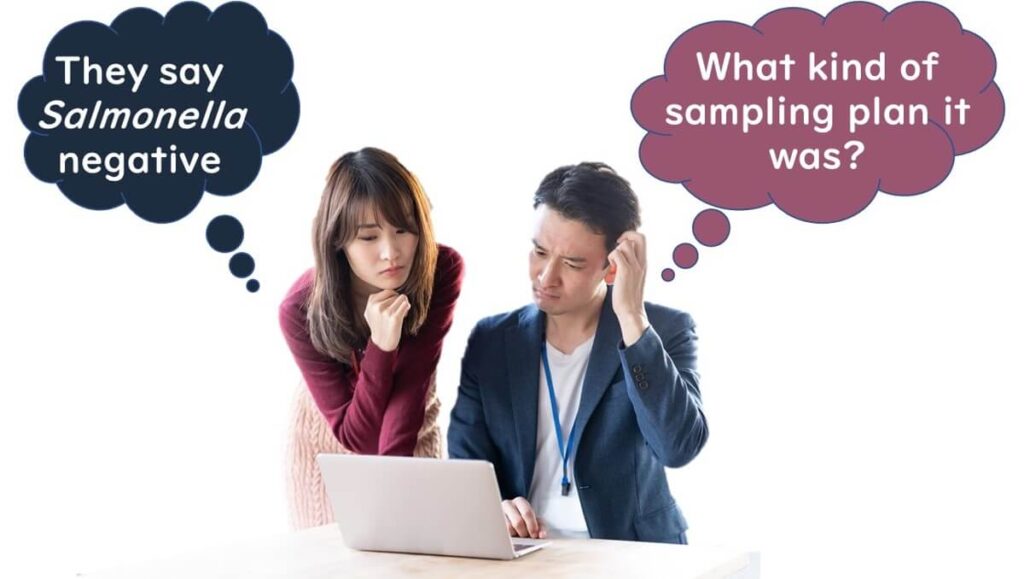
The interpretation of a negative result depends on the sampling plan used.
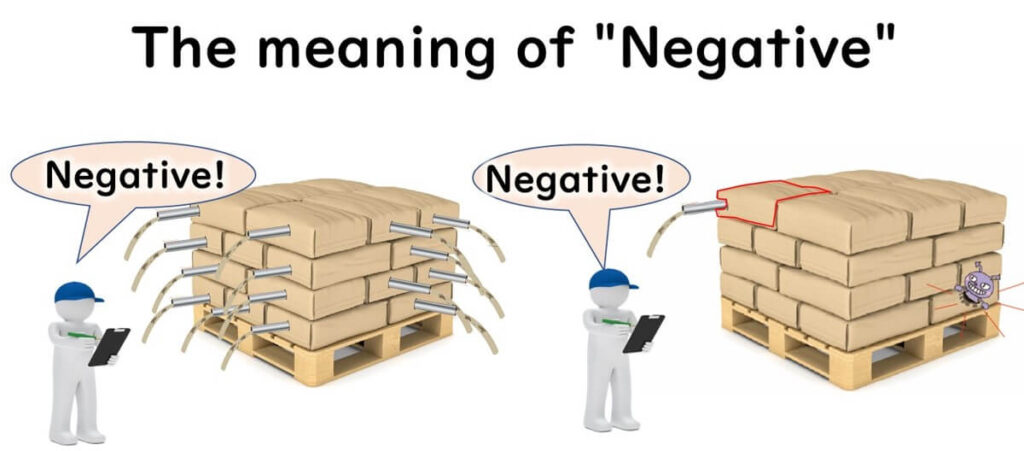
This article discusses the sampling plans adopted in the EU’s food safety and process hygiene standards. These plans are essentially based on those set by the International Commission on Microbiological Specifications for Foods (ICMSF).
For beginners in food microbiology, this article will first explain the key points essential to understanding these sampling plans.
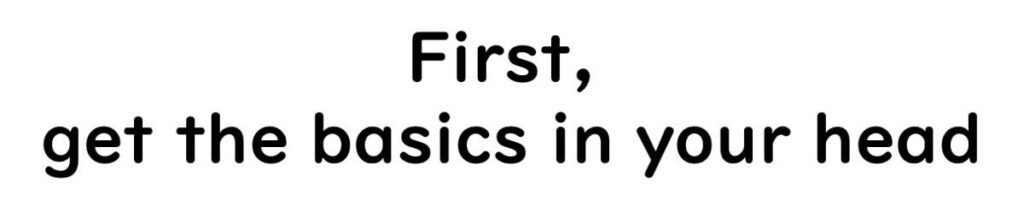
Types of ICMSF Sampling Plans and Their Purposes
ICMSF sampling plans can be broadly classified as follows:
- Two-class plan (Class 2): Primarily qualitative
- Three-class plan (Class 3): Quantitative
Note: Although some quantitative sampling plans are classified under the two-class plan, it is easier to understand the two-class plan as qualitative for now.
The microorganisms targeted in sampling tests are categorised as follows:
- Pathogenic bacteria like Salmonella (high risk)
- Indicator bacteria like E. coli (low risk)
Microbiological standards in the EU are broadly divided into:
- Food Safety Criteria: Non-compliance requires product recall
- Process Hygiene Criteria: Non-compliance requires a review of process control measures
For more detail, see the related article: “Differences in Microbiological Standards for Food in Japan and the EU, and Reasons for the Abolition of Hygiene Standards for Bento and Prepared Foods with HACCP Implementation.”
The relationship between sampling plans and these criteria can be summarised in the following table:
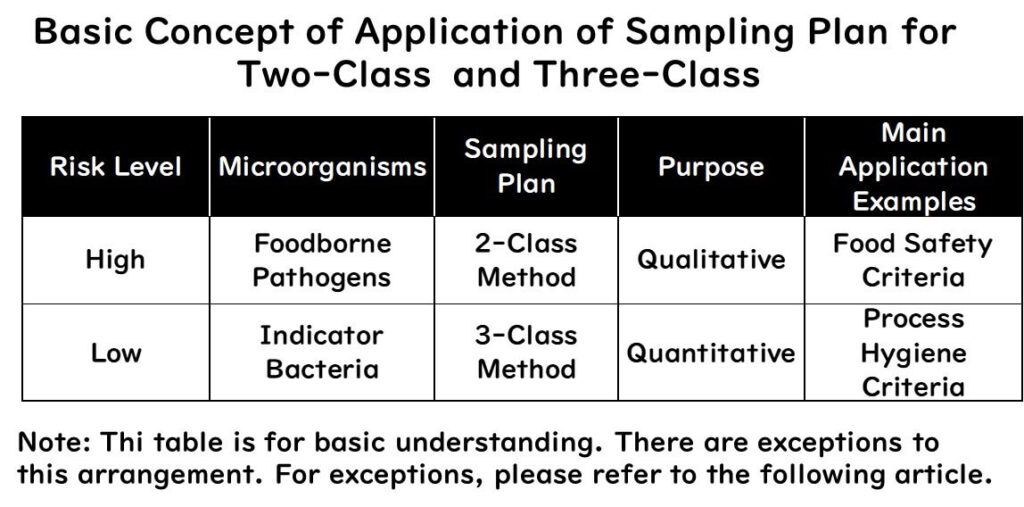
Reasons for the Above Summary
- High-risk pathogens like Salmonella must not be present in food. Therefore, qualitative assessment (positive or negative) is used, leading to a two-class sampling plan. This is primarily used in food safety criteria in the EU.
- Low-risk indicator bacteria are assessed quantitatively, meaning they must not exceed a certain amount. This leads to a three-class sampling plan, mainly used in process hygiene criteria in the EU.
Two-Class Sampling Plan (Qualitative)
A two-class sampling plan is a qualitative method that provides a binary result (presence or absence). This method is essential for pathogens that must not be present in food, such as foodborne illness-causing bacteria. Think of it as a red-card situation: a binary decision.
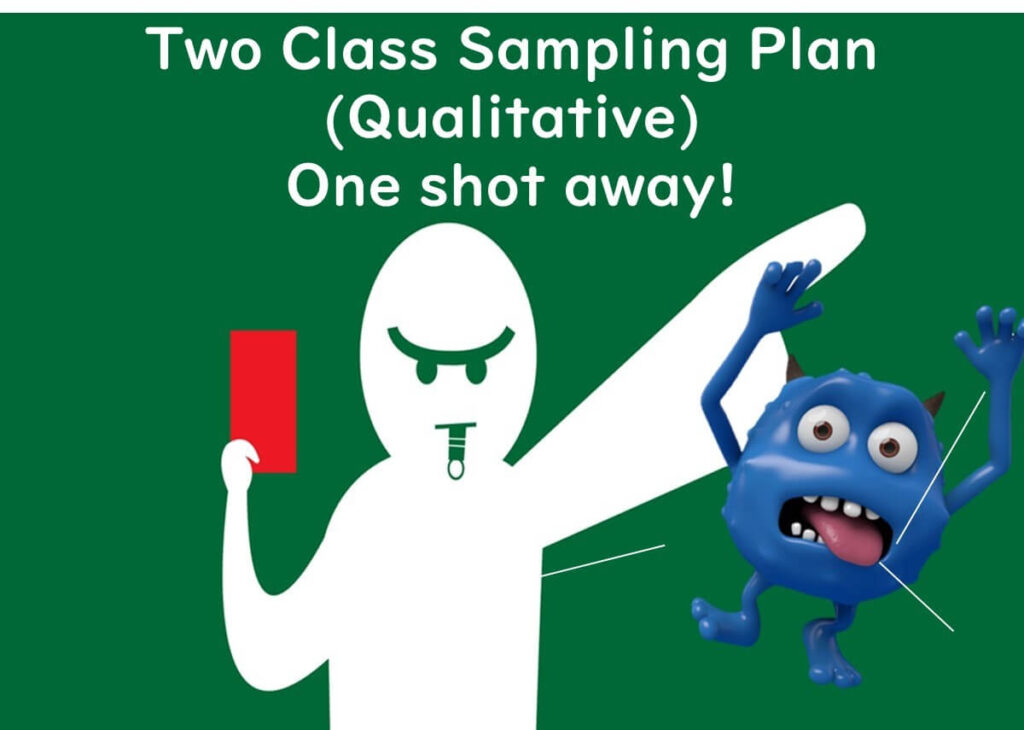
The diagram below shows the sampling plan for Salmonella in the EU’s food safety criteria for various products such as minced meat, raw poultry, egg products, cooked shellfish, and ice cream:
- n: Number of samples
- m: Safety standard value
- c: Acceptable number of positives
The following applies:
- Five samples must be tested (n = 5)
- No detection in 25g of the sample (m = 0/25g)
- Not a single sample exceeding the standard is acceptable (c = 0)
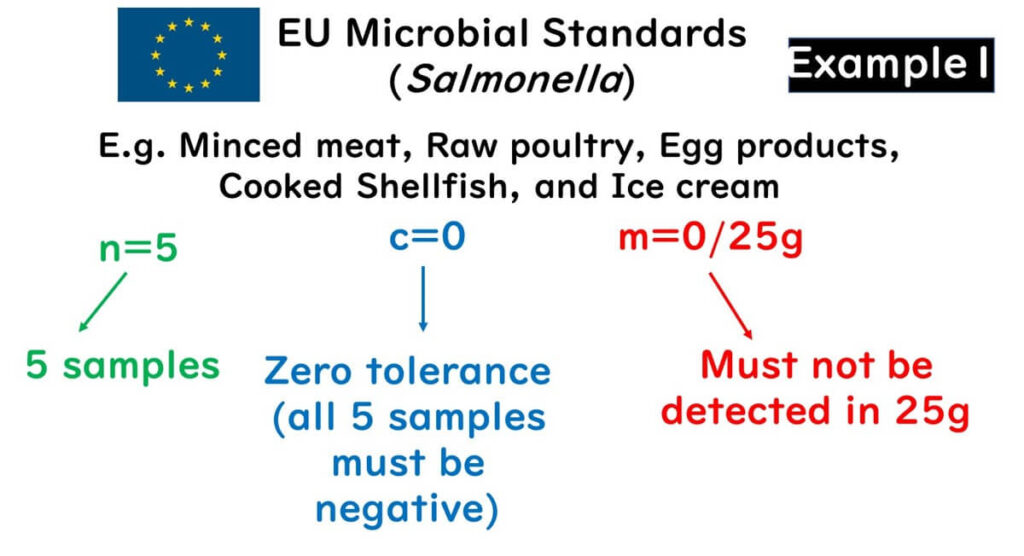
Most of the EU's food safety standards for Salmonella are set at n = 5.
Three-Class Sampling Plan (Quantitative)
While the two-class sampling plan involves a qualitative decision, the three-class sampling plan incorporates quantitative elements.
In the EU, this plan is used for indicator bacteria rather than pathogens and is applied in process hygiene criteria rather than food safety criteria.
Note: Exceptions exist.
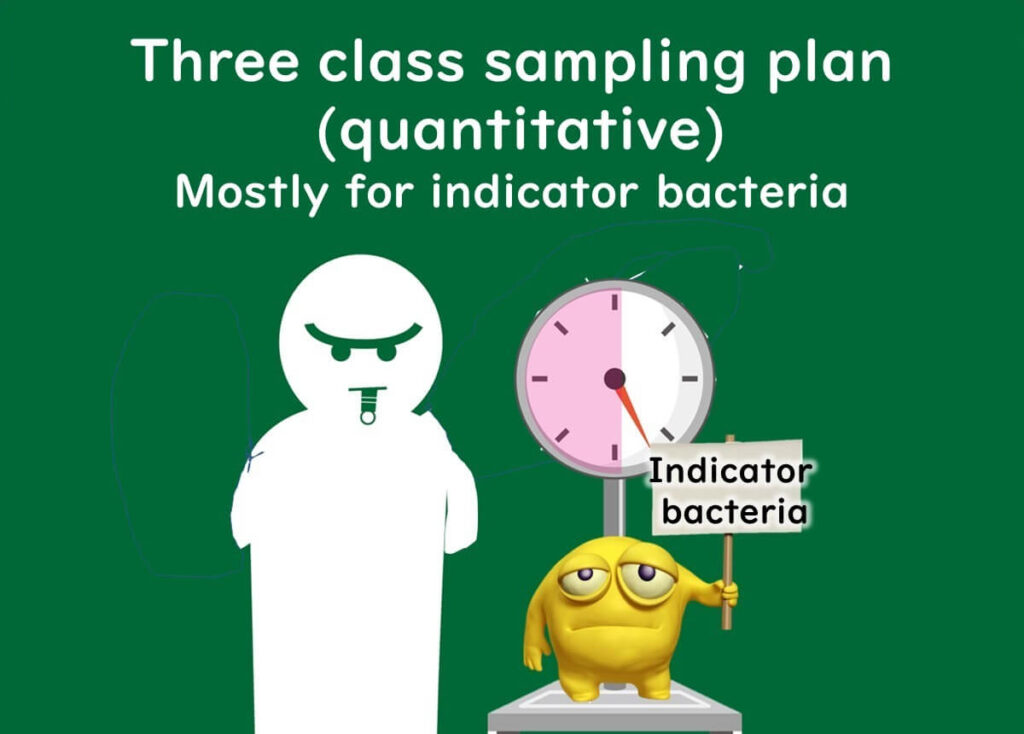
The diagram below shows the sampling plan for E. coli as an indicator bacterium in a cheese manufacturing plant under the EU’s process hygiene criteria. The plan is defined by (n, c, m, M).
For example:
- n = 5: Five samples need to be tested
- m = 100 cfu/g, c = 2: Up to two of the five samples can exceed 100 cfu/g
- M = 1000 cfu/g: If even one sample exceeds 1000 cfu/g, it is non-compliant
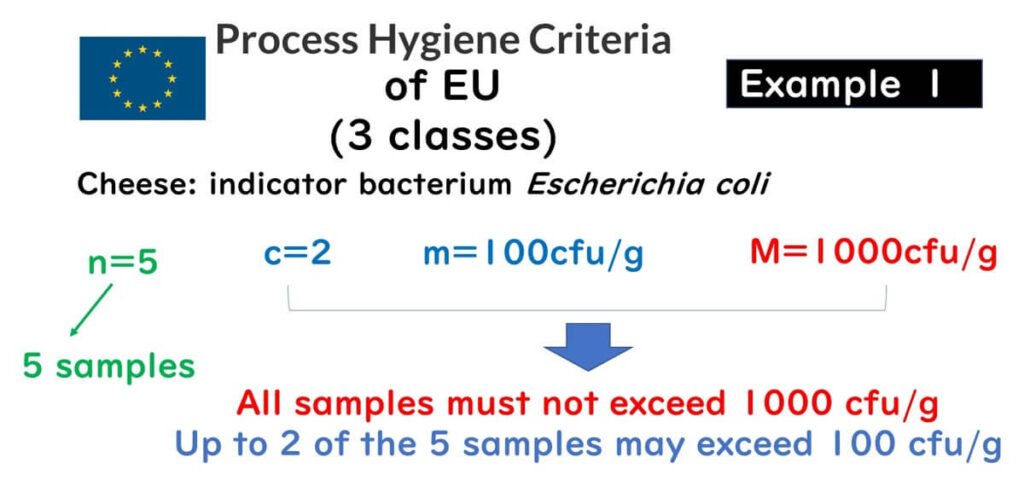
The diagram illustrates scenarios of pass and fail outcomes:
- Up to two out of five samples exceed 100 cfu/g, none over 1000 cfu/g → Pass
- One sample exceeds both 100 cfu/g and 1000 cfu/g → Fail
- Three samples exceed 100 cfu/g, regardless of 1000 cfu/g → Fail
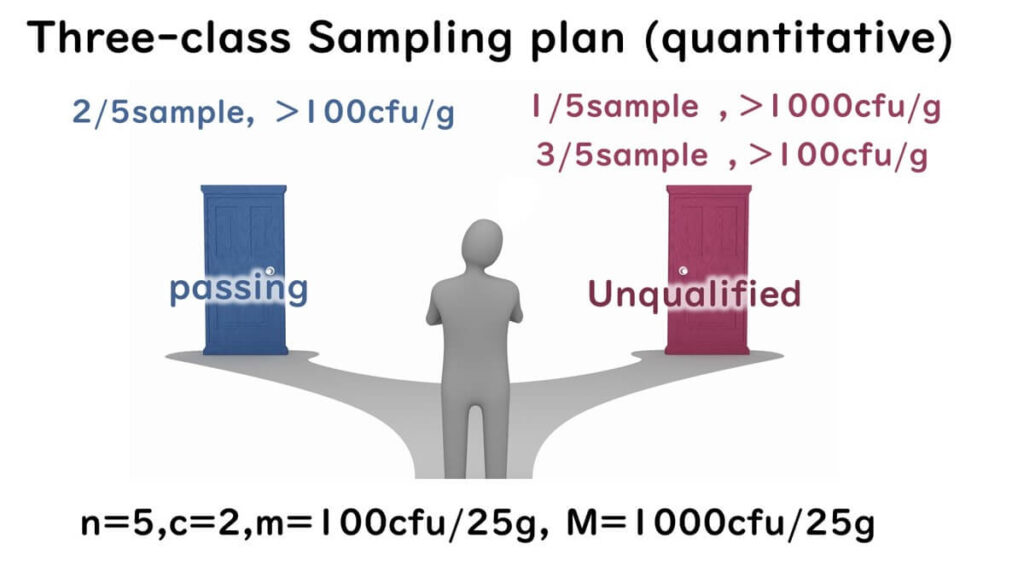
Sampling Plan Design Strategy
The table below provides general guidelines for which sampling plan to use based on microorganisms and food types.
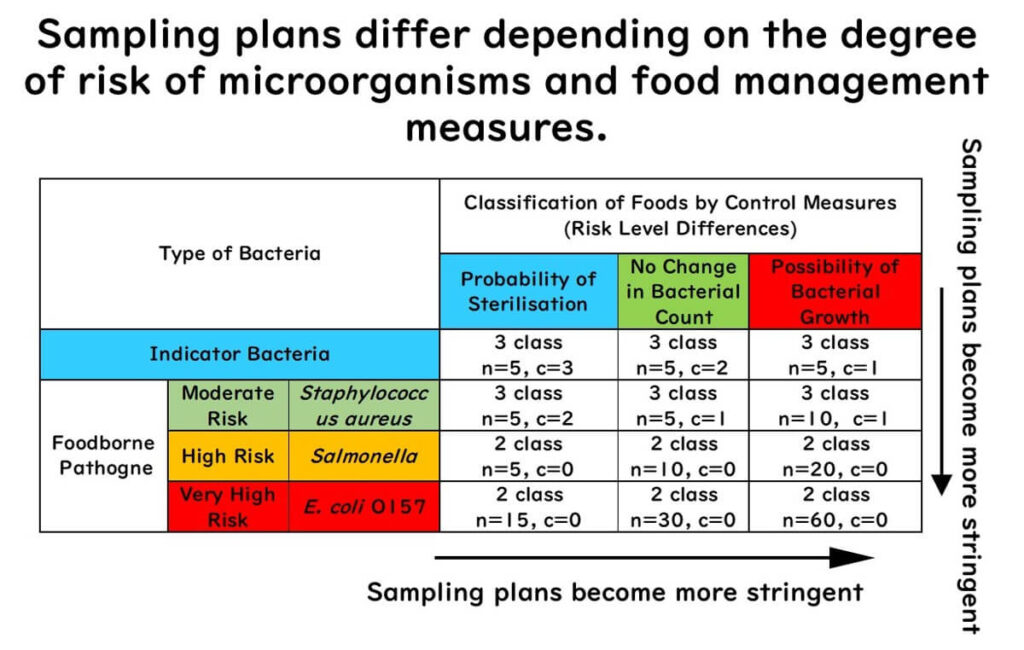
The stringency (detection sensitivity) of a sampling plan is determined by several factors:
- Two-class plans are stricter than three-class plans, with no tolerance for deviation
- In any plan, increasing n and decreasing acceptable positives (c) increases stringency
- Microorganisms vary in risk:
- Low (indicator bacteria)
- Moderate (e.g. Staphylococcus aureus)
- High (e.g. Salmonella)
- Very high (e.g. E. coli O157)
- Food management depends on whether food can be sterilised and whether bacteria may multiply during distribution
Strategy Summary
- As microorganism risk increases, the sampling plan becomes stricter
- Foods that can be sterilised may have more lenient plans; if bacterial growth is possible during distribution, stricter plans are needed
Accuracy of Sampling Plans
How accurate are these sampling plans?
Example 1: Salmonella Sampling Plan with n = 5 in the EU
The chart below shows the probability of detecting various Salmonella contamination rates when testing five samples.
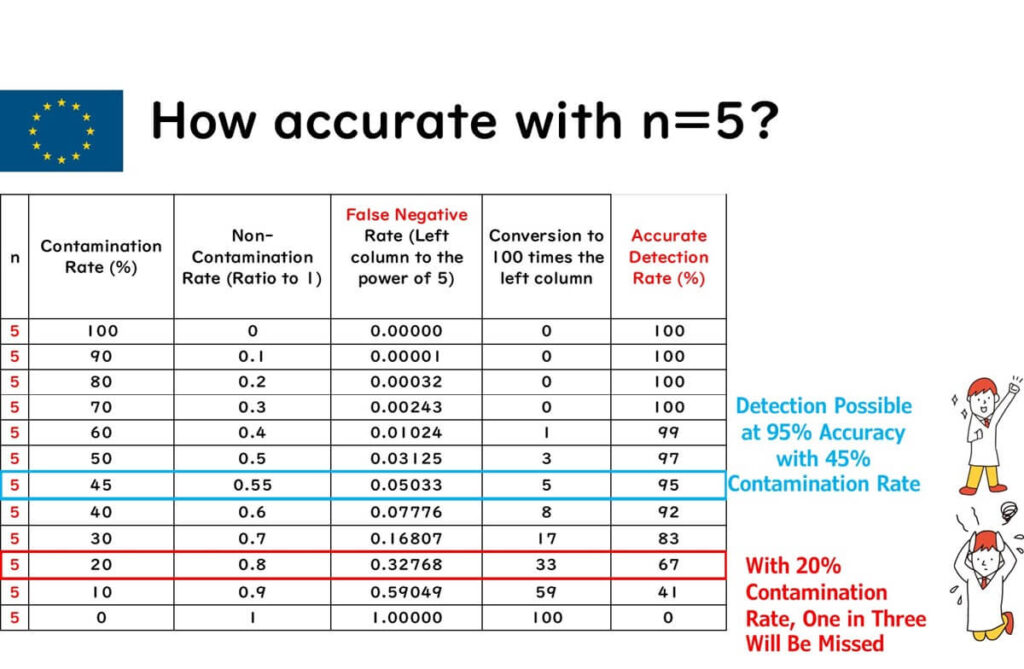
For beginners, a separate article includes a step-by-step explanation and video guide on the creation of this table and calculation of testing accuracy.
From the chart:
- At a 45% contamination rate, detection probability is 95%
- At a 20% contamination rate, detection drops to 67% — one in three tests could miss it (false negative)
Example 2: Salmonella Sampling Plan with n = 30 for Infant Formula
For infant formula in the EU:
- n = 30
- No detection in 25g (m = 0/25g)
- No positives allowed (c = 0)
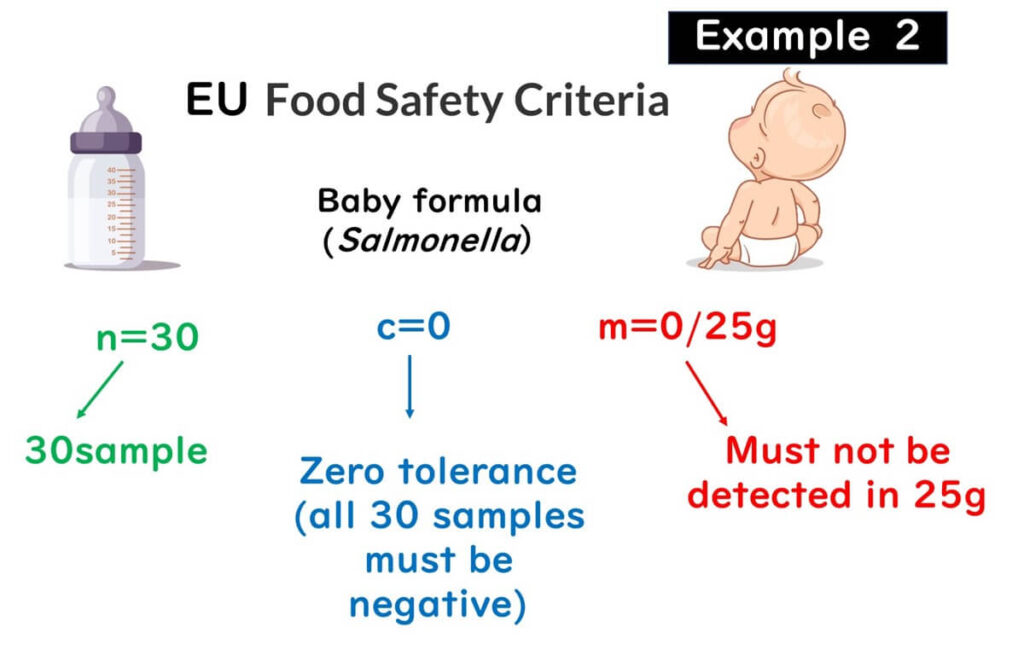
The ICMSF recommends n = 60, but EU uses n = 30.
The chart below shows detection probability for different contamination rates with n = 30.
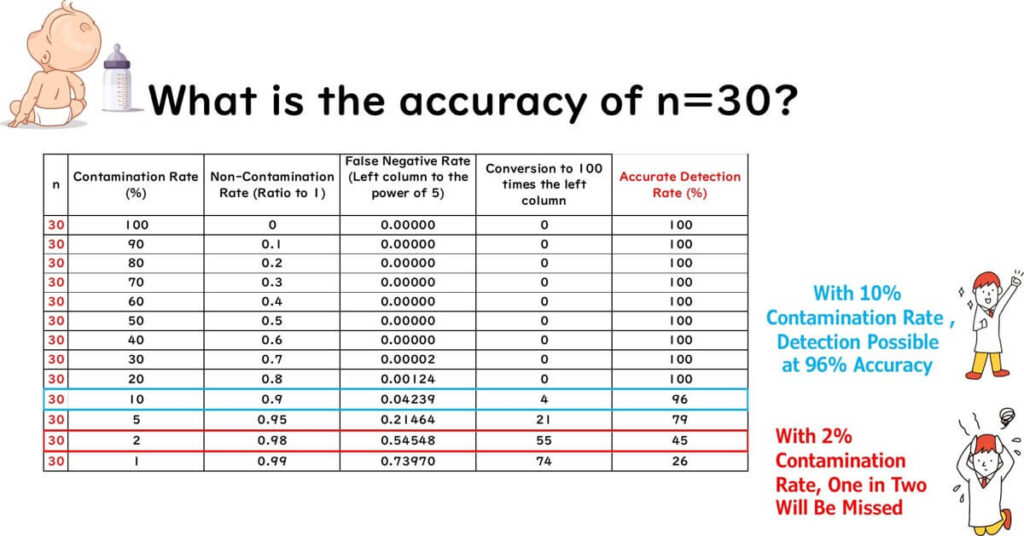
From the chart:
- At 10% contamination rate, detection probability is 96%
- At 2% contamination rate, detection probability drops to 45% (1 in 2 false negative)
Relationship Between Sampling Plans and Food Safety Evaluation
As discussed, increasing the sample number n cannot eliminate all risk. Without testing every unit, 100% certainty is unachievable.
So, are these sampling plans still meaningful?
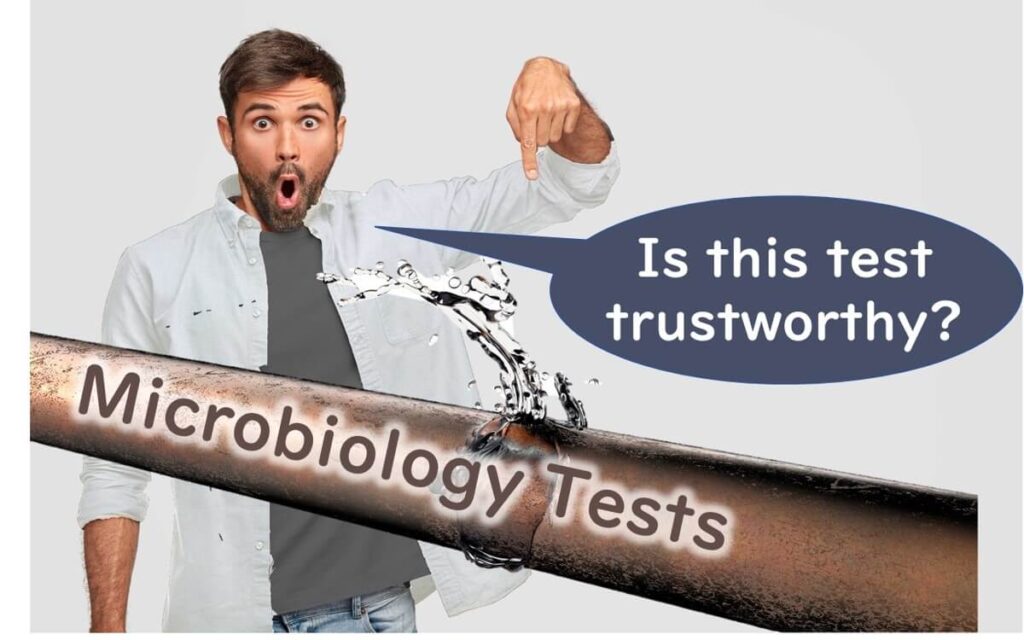
Yes.
- A properly designed sampling plan allows reasonable estimation of microbial concentration (microbial load)
Lower Contamination Rates Indicate Lower Microbial Load
There is a correlation between contamination rate and microbial load:
- Lower contamination rate → lower average microbial concentration
Think of it as a mountain: a narrow base suggests a small hill; a wide base may mean a towering peak like Mount Fuji.
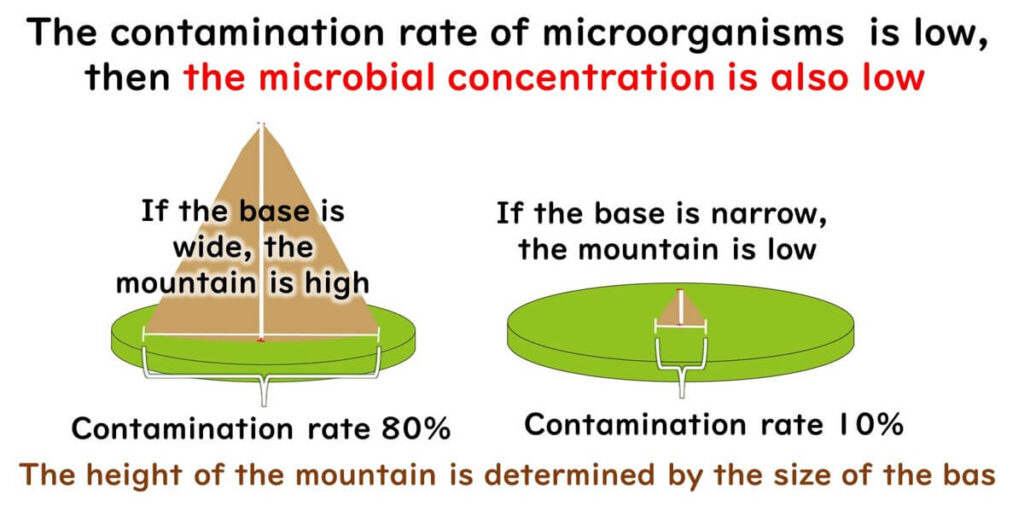
To explain further, consider Russian Roulette:
- One bullet (10%) or ten bullets (100%) — either way, pulling the trigger can be fatal
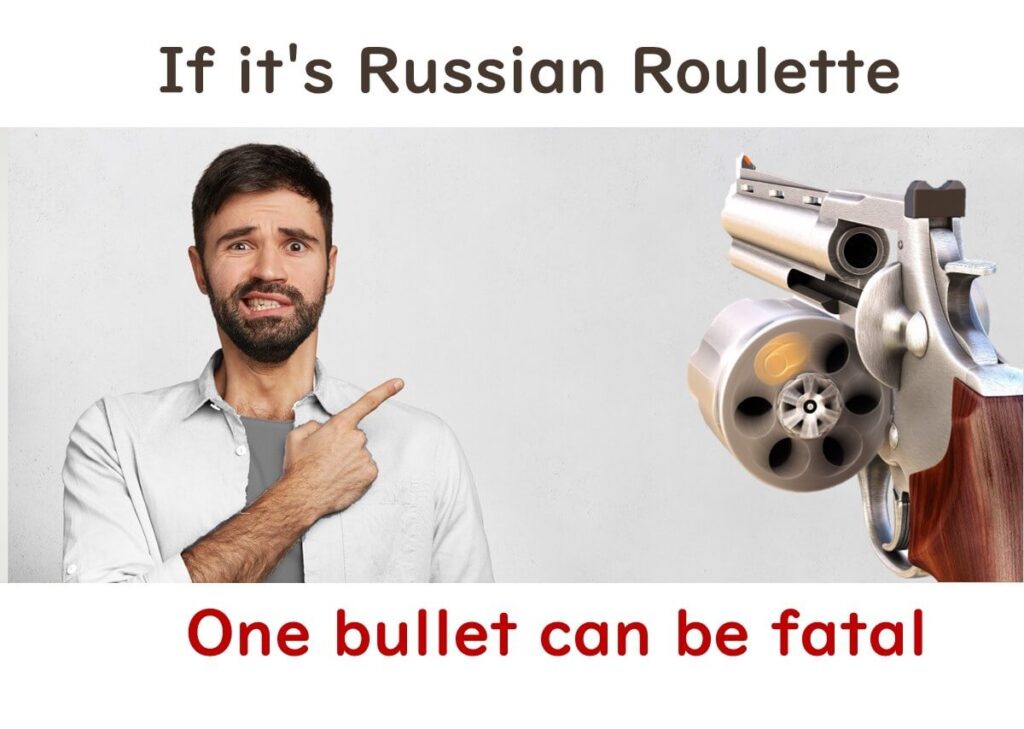
But microbiological testing isn’t Russian Roulette.
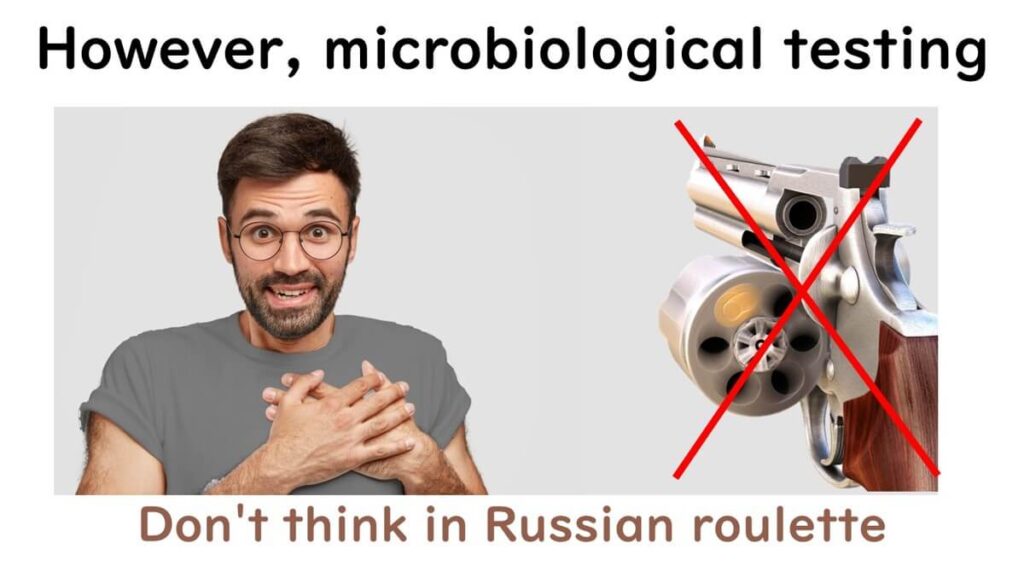
In food testing, fewer "bullets" (lower contamination rate) mean even if hit (positive result), the impact (concentration) may be minimal.
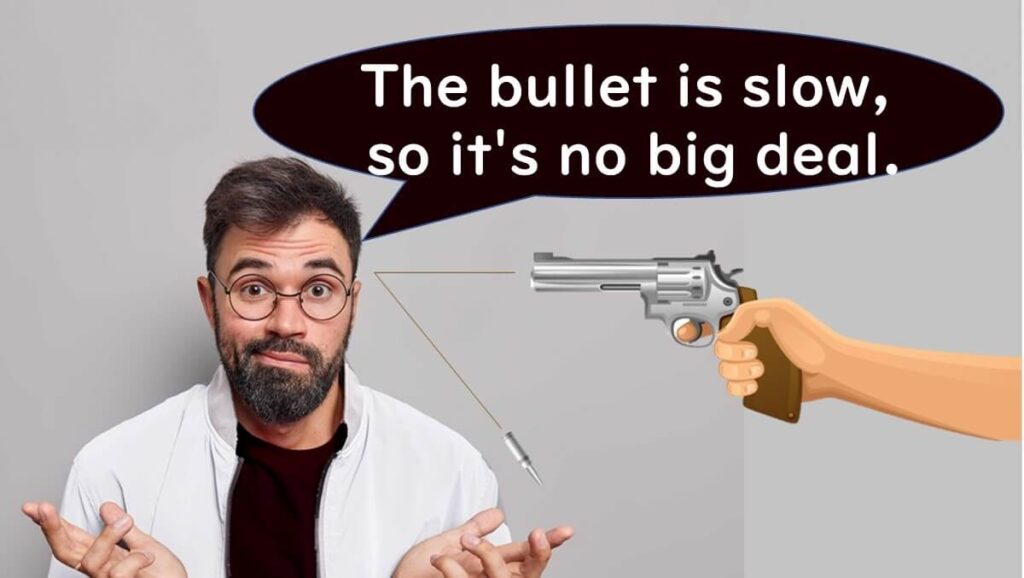
Why?
Microorganism distribution in food often follows a log-normal distribution:
- When graphed on a logarithmic scale, the curve is symmetrical
- The highest probability lies at the mean
- Probability decreases as values diverge from the mean
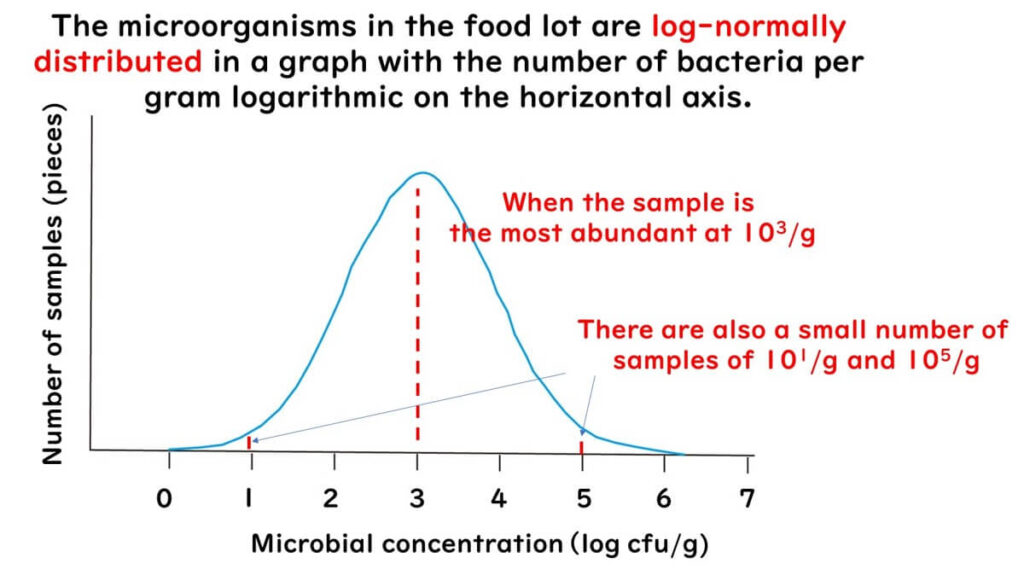
For example, with a criterion of 10³/g:
- If 50% of samples pass, the mean is 10³/g
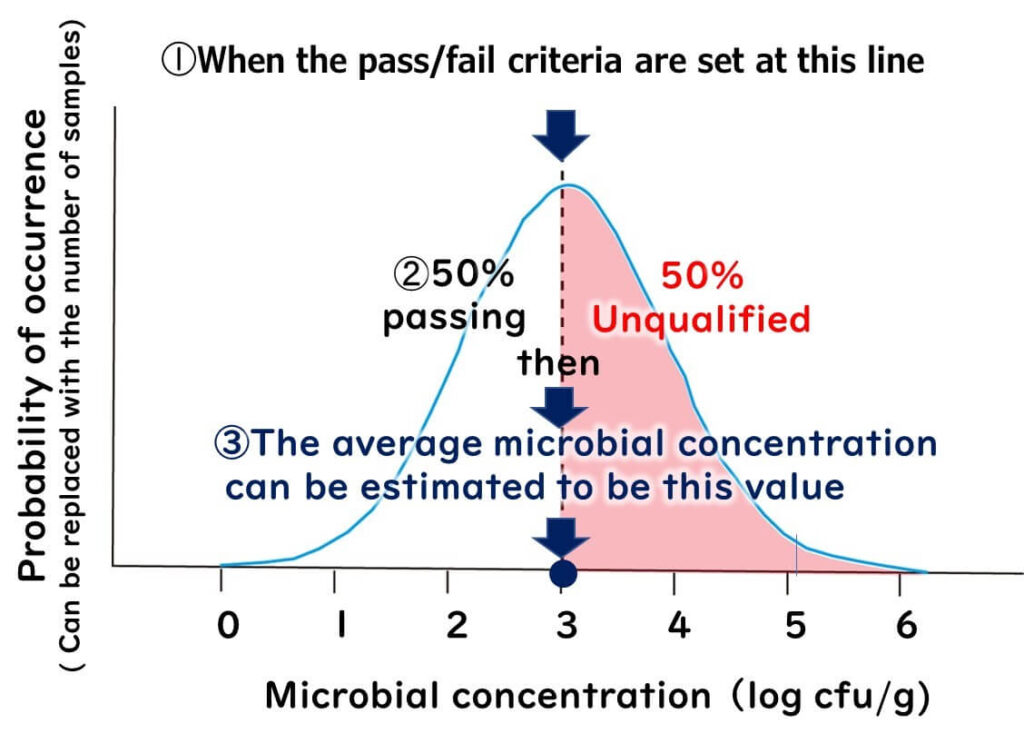
If 20% fail and 80% pass:
- The mean shifts left to ~1.7 log = ~5×10¹/g
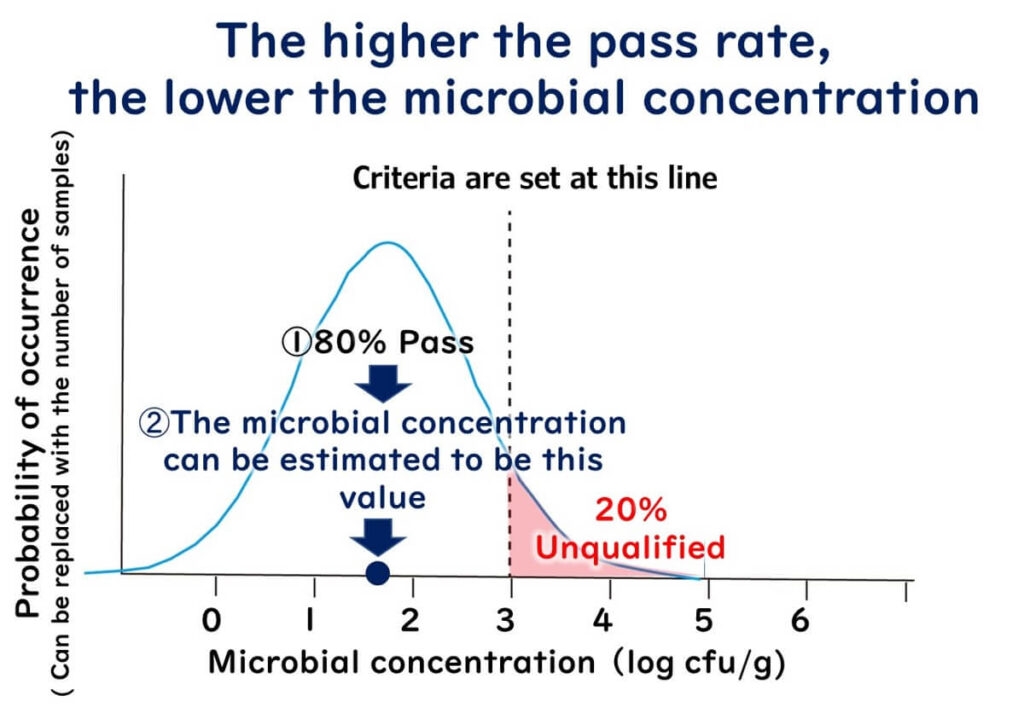
Conclusion from the Above:
- Lower contamination rate → lower average microbial concentration
- Higher contamination rate → higher average microbial concentration
Estimating Contamination Levels from Contamination Rates
By applying the above principles, one can estimate microbial concentrations based on contamination rates.
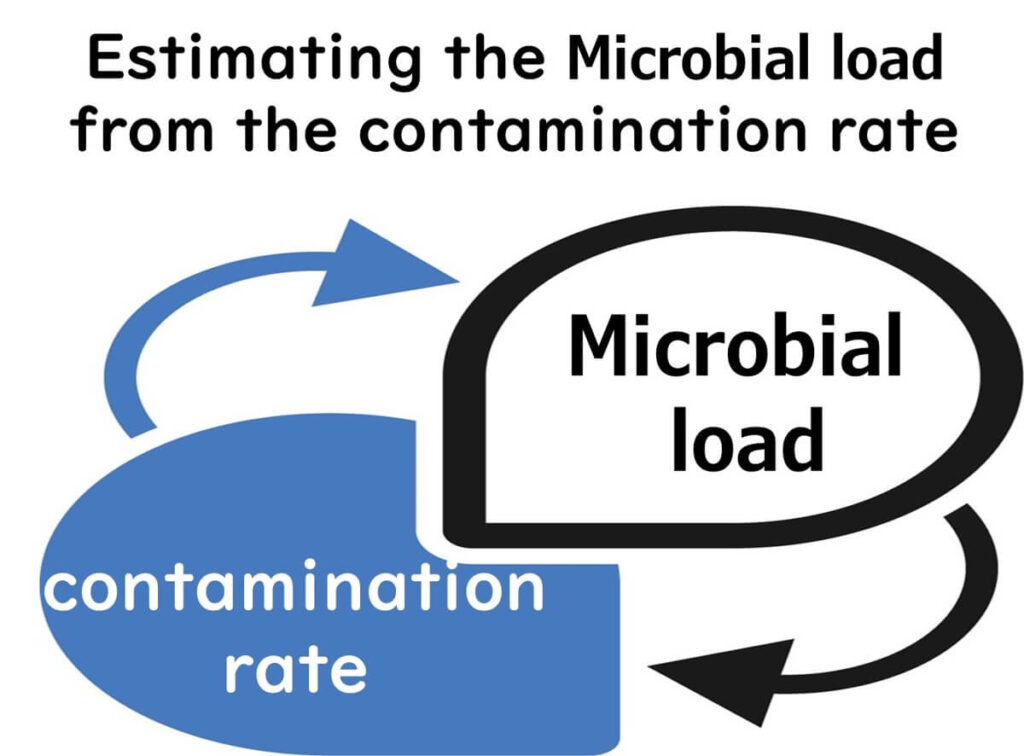
However, estimation depends on the standard deviation, which defines the shape of the distribution.
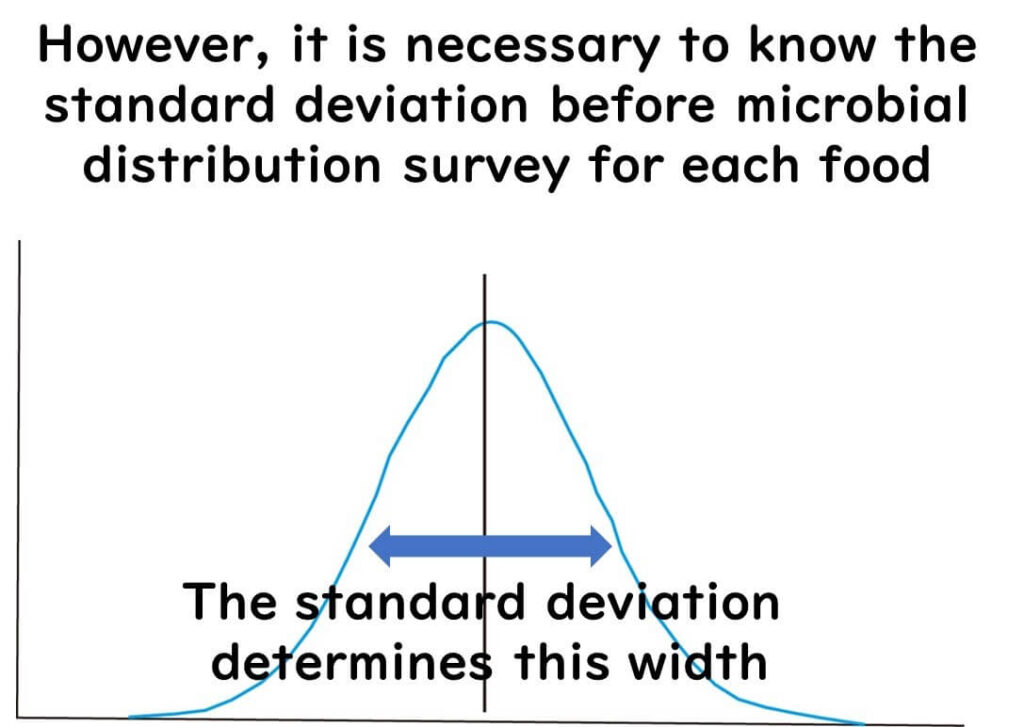
Standard deviation must be measured for each food type — it varies by food, microorganism, and process.
Once standard deviation is known, contamination rate from sample results can predict average microbial concentration for the batch.
If no prior data exists, a default standard deviation value can be used.
This chart shows how a 30%, 50%, or 70% positive rate converts into average microbial concentrations (assuming a criterion of 10³ log cfu/g):
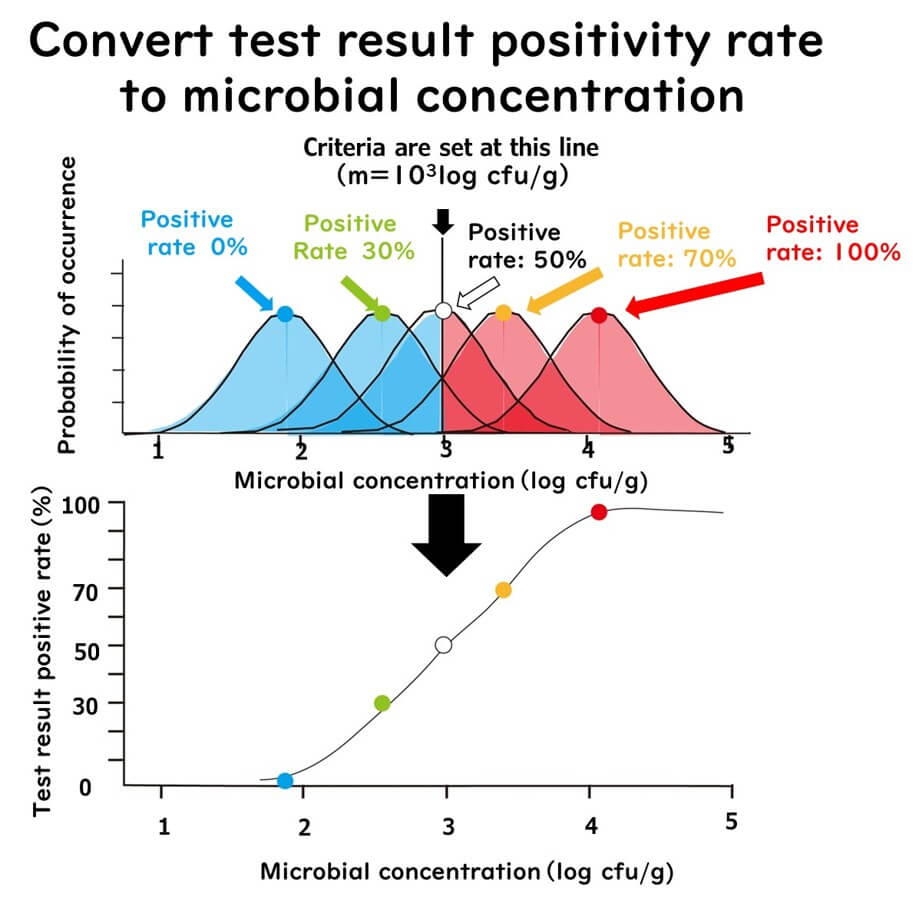
- 30% positive → ~10².⁷ log cfu/g
- 50% positive → ~10³.⁰ log cfu/g
- 70% positive → ~10³.⁵ log cfu/g
Using this, the ICMSF provides expected microbial concentrations for various sampling plans at 95% detection probability:
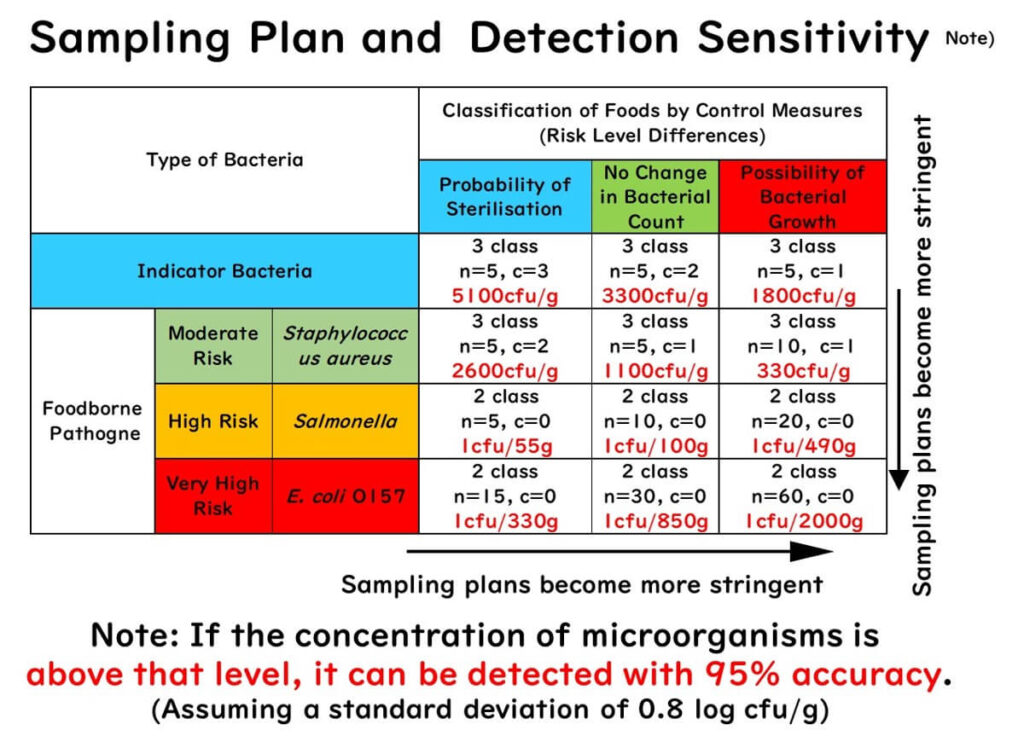
This article does not cover the exact formulas. Understanding the concept is sufficient for most food microbiology practitioners.
Summary for Beginners
To summarise:
- Even with a large number of samples, some contamination may be missed
- However, based on sampling plan accuracy, one can estimate microbial concentrations at a 95% detection level
- By selecting appropriate sampling plans tailored to the microorganism and food type, food safety risks can be effectively managed
Once these points are grasped, beginners will have a strong foundation.
Additional Information on ICMSF Sampling Plans
This section is for readers who understand the basics covered earlier.
ICMSF sampling plans include more than just the two described previously.
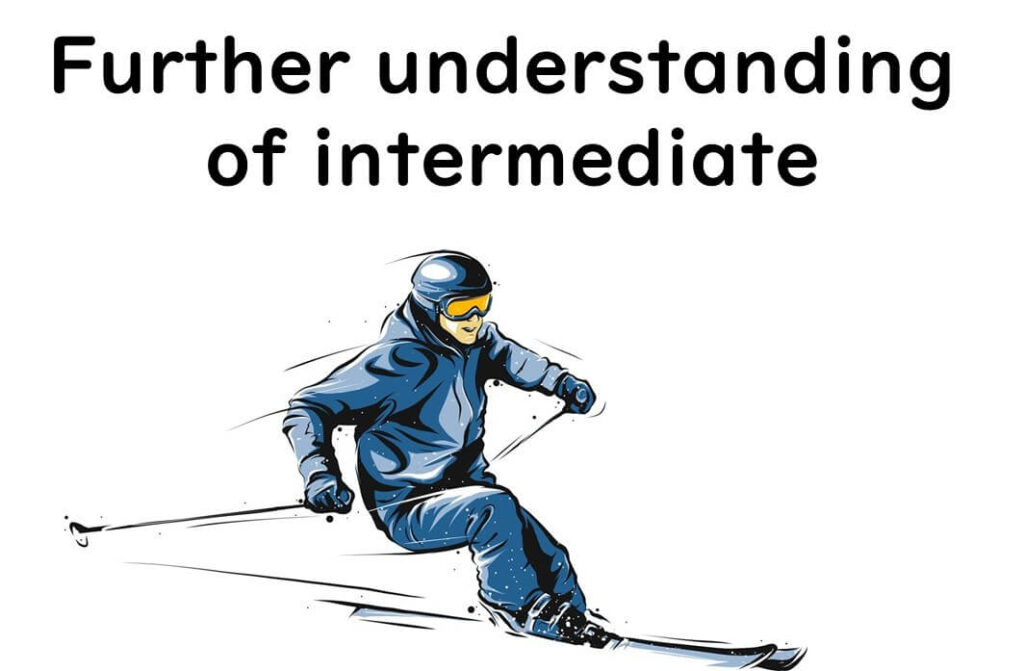
Quantitative Two-Class Sampling Plan
Although two-class plans are often qualitative, ICMSF also defines quantitative two-class plans.
Example: EU standards for Listeria in ready-to-eat foods (where Listeria cannot grow during distribution):
- Up to 100 cfu/g is allowed
Foods where Listeria can grow are held to zero tolerance (non-detectable in 25g)
For Listeria in non-growth environments:
- n = 5, m = 100 cfu/g, c = 0
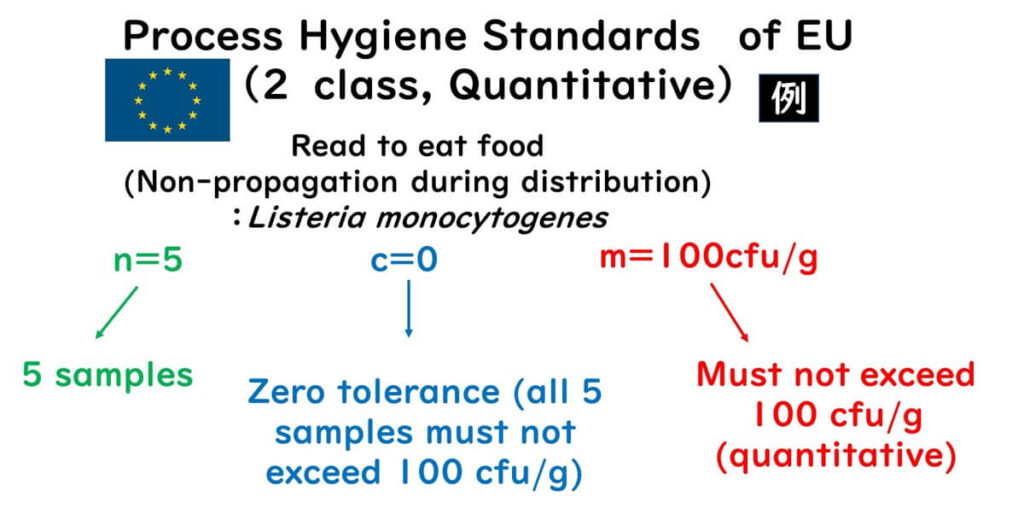
Comparison:
- Qualitative: non-detectable in 25g
- Quantitative: limit of 100 cfu/g
Hence, this is termed a quantitative two-class sampling plan.
"Qualitative + Quantitative" Mixed Sampling Plan
The mixed three-class sampling plan combines both approaches. Proposed by ICMSF in 2020 for Listeria in ready-to-eat foods.
For details, see Marcel H. Zwietering’s ICMSF 2020 video presentation.
Summary:
- n = 5
- m = 0/25g (Listeria must not be detected)
- c = 1 (1 sample exceeding allowed)
- M = 100 cfu/g: if any sample exceeds this, it fails
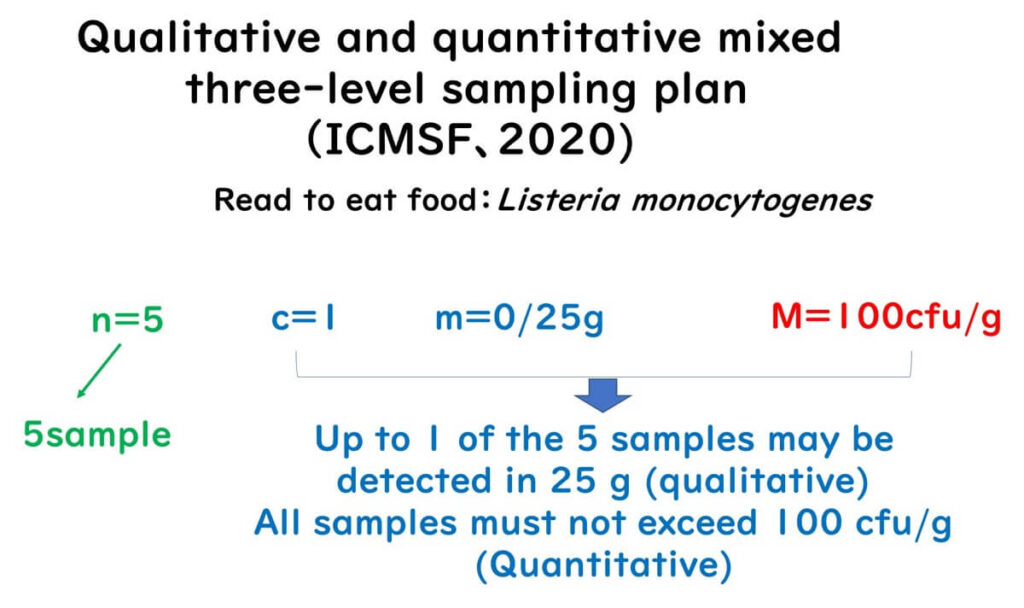
Why Introduce This Plan?
Reference: Farber et al., Food Control, May 2021
The zero-tolerance two-class plan has drawbacks:
- Frequent recalls and economic losses
- Manufacturers may avoid voluntary testing due to fear of recalls
The mixed plan allows flexibility while maintaining safety:
- Detection sensitivity remains high, often stricter than zero-tolerance plans in the US (n = 1 or 2)
Conclusion
Sampling plans in food microbiology are essential. Understanding the basic principles, especially the rationale behind them, is critical for beginners.
However, detailed calculations are not required for most practitioners. Understanding the direction and purpose of sampling plans is sufficient; technical computations can be left to experts.
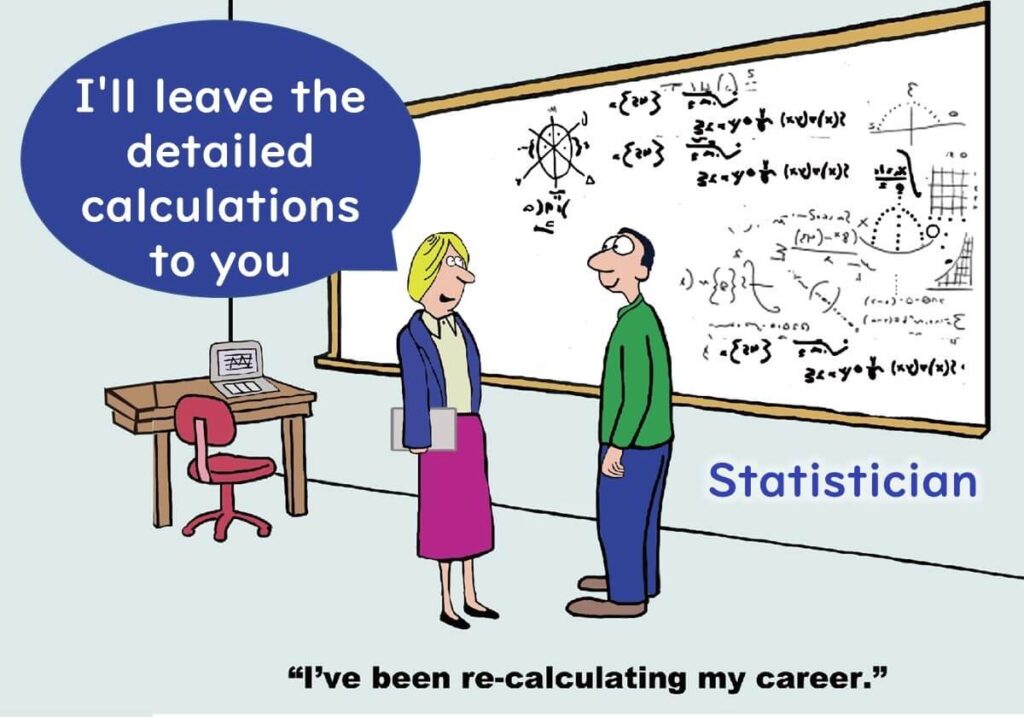
For more information on ICMSF sampling plans, visit the ICMSF website, which offers books and educational videos.